At A Heart 2 Help, we believe in the power of technology to create fair and equitable digital spaces. However, this vision can only be realized through the development and implementation of inclusive algorithms.
These algorithms play a crucial role in shaping our online experiences, from social media feeds to job application processes. Yet, many current algorithms perpetuate biases and discrimination, highlighting the urgent need for more inclusive approaches in AI and machine learning.
Why Inclusive Algorithms Are Essential for Fair Digital Spaces
The Foundation of Equitable Online Experiences
Inclusive algorithms form the cornerstone of fair digital spaces. These algorithms treat all users equally, regardless of race, gender, age, or other characteristics. They provide unbiased results and experiences for everyone who interacts with digital platforms.
Real-World Consequences of Algorithmic Bias
The impact of biased algorithms extends far beyond the digital realm. In 2015, Google’s image recognition algorithm mislabeled photos of Black people as gorillas, underscoring the urgent need for more diverse training data. This incident not only caused user distress but also tarnished the company’s reputation.
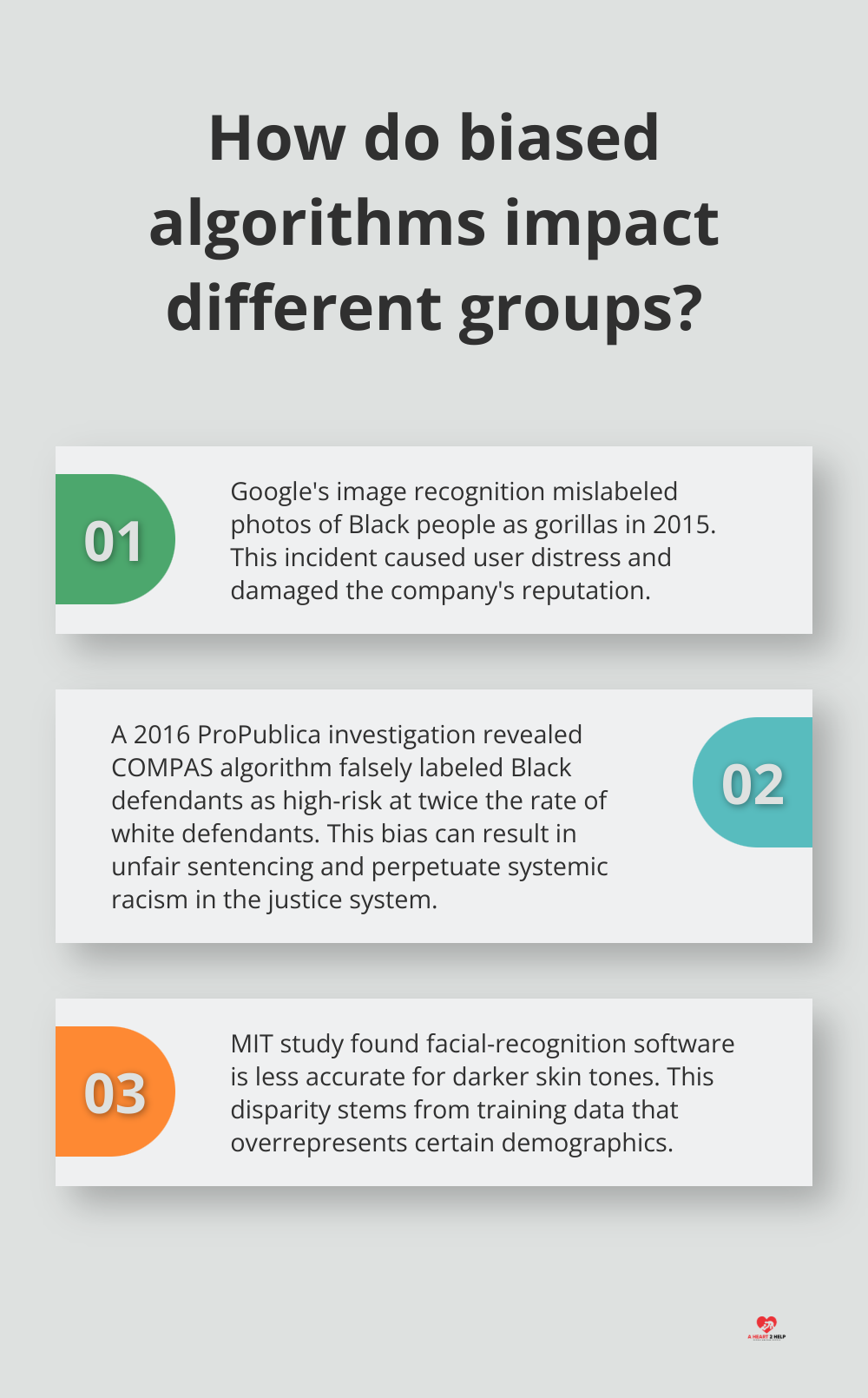
The criminal justice system offers another stark example. A 2016 ProPublica investigation revealed that the COMPAS algorithm (used to predict recidivism rates) falsely labeled Black defendants as high-risk at nearly twice the rate of white defendants. Such bias can result in unfair sentencing and perpetuate systemic racism in the justice system.
Shaping User Experiences Through Inclusive Algorithms
Inclusive algorithms have the power to create more equitable digital spaces. Unbiased algorithms in job search platforms can help reduce discrimination in hiring processes. LinkedIn, for instance, has implemented measures to ensure their job recommendation systems don’t perpetuate gender biases in certain industries.
The Critical Role of Diverse Data
The development of inclusive algorithms starts with diverse data sets. A study by Joy Buolamwini at MIT found that facial-recognition software is less accurate when identifying darker skin. This disparity stems from training data that overrepresents certain demographics.
To address this issue, companies must actively seek out diverse data sources. Google has launched initiatives (like their Inclusive Images Competition) to encourage the development of more representative image recognition models.
The Path Forward: Collaboration and Innovation
The creation of truly inclusive algorithms requires collaboration across disciplines and industries. Tech companies, researchers, and policymakers must work together to identify and address biases in AI systems. This collaborative approach (combined with ongoing innovation) will pave the way for more equitable digital spaces that serve all users fairly.
As we move forward, it’s essential to recognize the challenges that come with developing inclusive algorithms. Let’s explore these obstacles and the strategies needed to overcome them in our pursuit of fair digital spaces.
Overcoming Hurdles in Inclusive Algorithm Development
The Data Dilemma
Algorithm development relies on data, but this data often mirrors societal biases and historical inequalities. A 2018 study by the AI Now Institute exposed a stark disparity in facial recognition systems: error rates reached up to 34.7% for darker-skinned women, compared to a mere 0.8% for lighter-skinned men. This gap results from training datasets that overrepresent certain demographics while underrepresenting others.
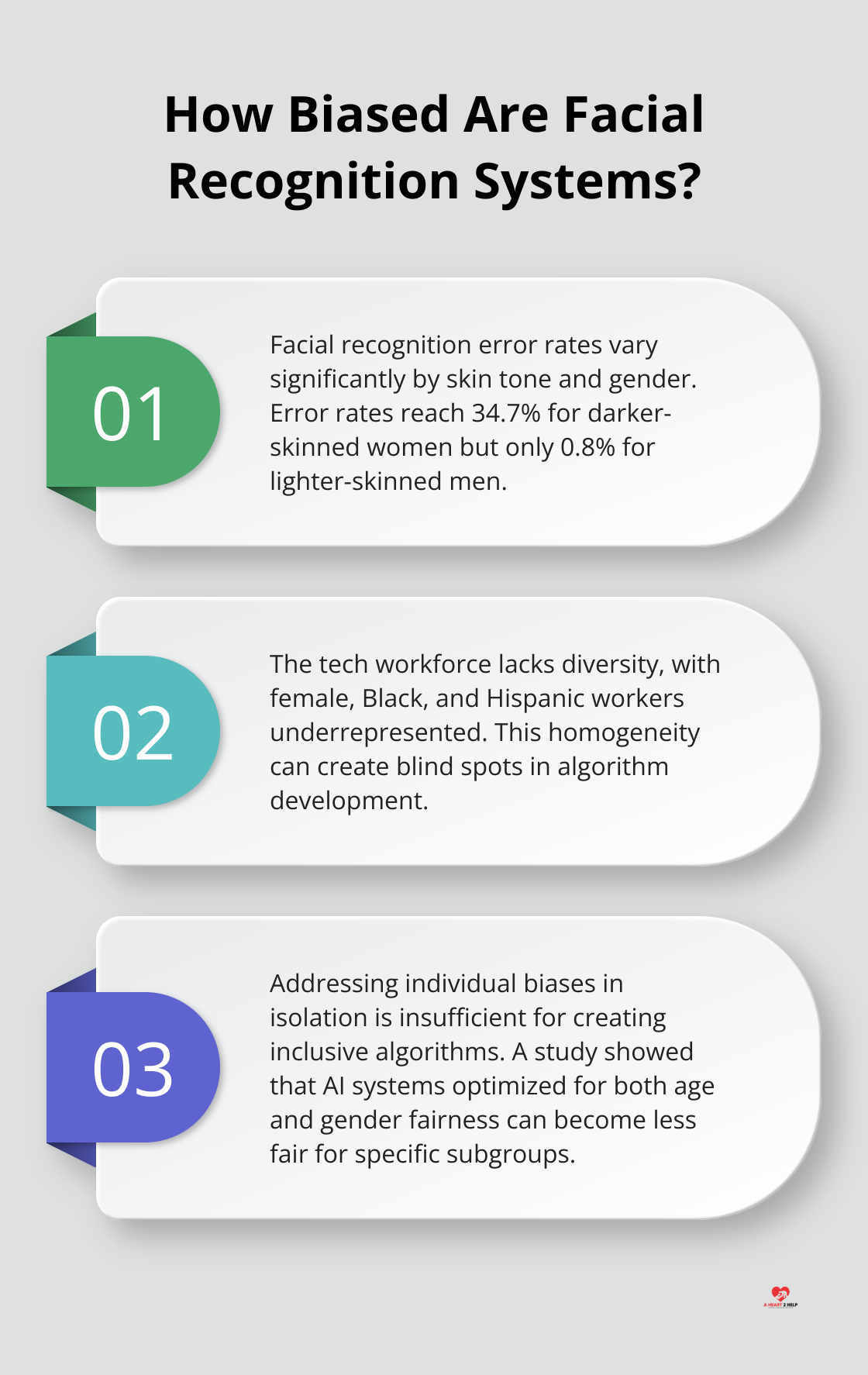
To tackle this issue, companies must collect and curate diverse, representative datasets. IBM has taken steps in this direction with their Diversity in Faces dataset (which aims to improve facial recognition accuracy across different demographics).
Diversity Deficit in Tech
The tech workforce’s lack of diversity compounds the challenge of creating inclusive algorithms. Female, Black, and Hispanic workers remained substantially underrepresented in the high tech workforce and sector between 2005 and 2022. This homogeneity can create blind spots in algorithm development, as teams may overlook biases that don’t affect them personally.
Increasing diversity in tech extends beyond hiring practices. It requires the creation of inclusive work environments where diverse perspectives shape decision-making processes. Intel, for example, has set ambitious diversity goals (aiming for full representation of women and underrepresented minorities in their U.S. workforce by 2030).
Tackling Multiple Biases Simultaneously
Algorithms interact with complex social systems that harbor multiple, intersecting forms of bias. A 2019 study published in the Proceedings of the National Academy of Sciences revealed an unexpected challenge: an AI system trained to be fair with respect to both age and gender ended up less fair for older women and younger men than a system optimized for only one type of fairness.
This complexity demands a holistic approach to algorithm development. Addressing individual biases in isolation isn’t enough; we must consider how different forms of bias interact and impact various user groups. Tools like IBM’s AI Fairness 360 toolkit provide resources for detecting and mitigating multiple types of bias in machine learning models.
The journey to truly inclusive algorithms presents significant challenges, but it’s a path we must follow to create fair digital spaces. As we confront these obstacles, the next section will explore strategies that tech companies, researchers, and diverse communities can employ to develop solutions that serve all users equitably.
How to Build Truly Inclusive Algorithms
Creating inclusive algorithms requires a multifaceted approach. Tech companies and developers can implement several practical strategies to create algorithms that serve all users fairly:
Diversify Data Collection and Curation
The foundation of any inclusive algorithm lies in its training data. Companies must actively seek out diverse datasets that represent a wide range of demographics, experiences, and perspectives. Strategies include collecting diverse and representative data, using bias-aware algorithms, enhancing model interpretability, and applying pre-processing techniques.
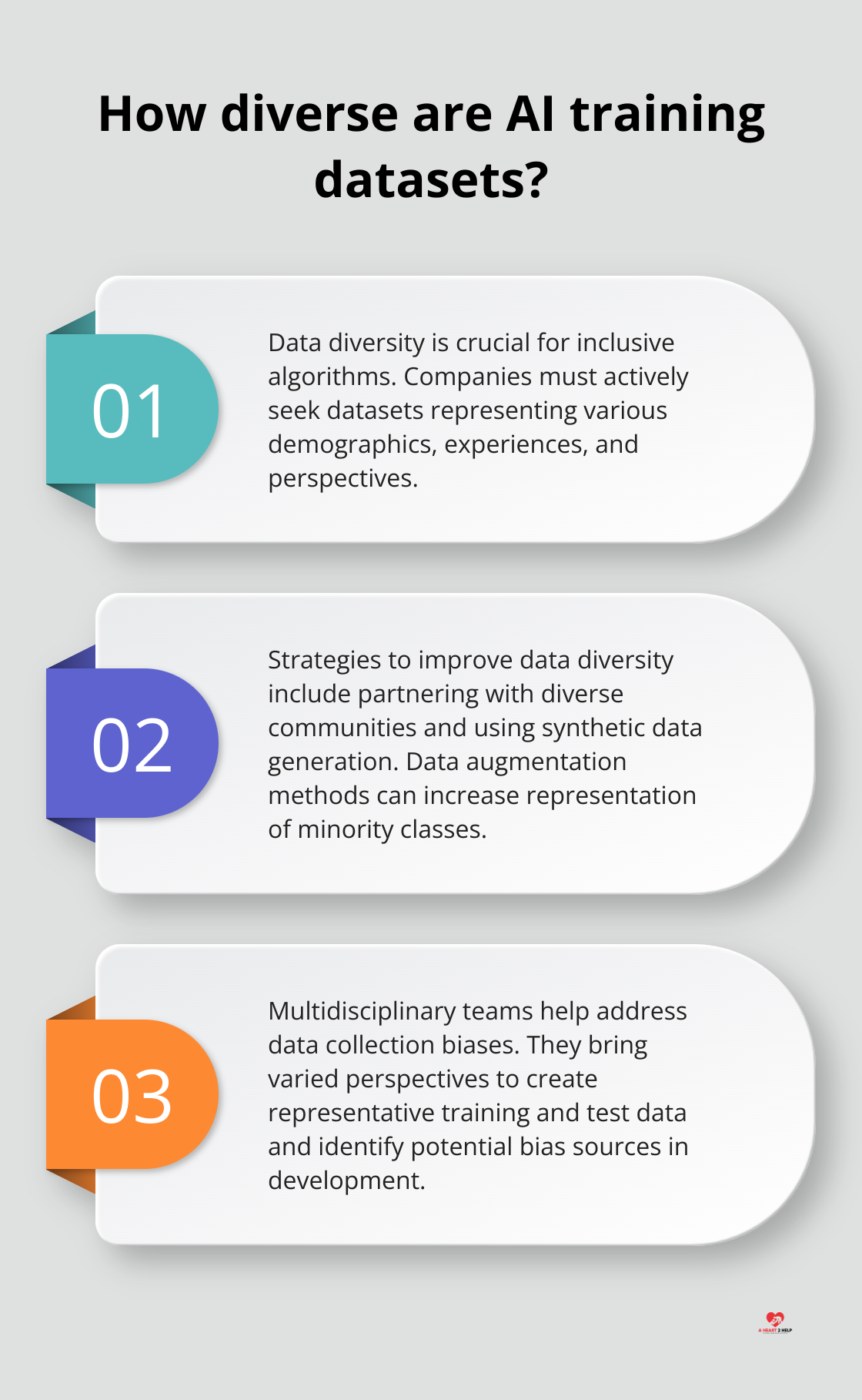
To improve data diversity, organizations can:
- Partner with diverse communities to gather representative data
- Use synthetic data generation techniques to fill gaps in underrepresented groups
- Implement data augmentation methods to increase representation of minority classes
Foster Interdisciplinary Collaboration
Algorithm development should not limit itself to computer scientists and engineers. Bringing together experts from various fields helps identify and address potential biases that may not appear obvious to those with purely technical backgrounds.
A multidisciplinary team can help address data collection biases by bringing an array of perspectives to creating representative training and test data and identifying potential sources of bias in the development process.
Implement Regular Audits and Bias Testing
Continuous monitoring and testing of algorithms for bias proves essential. Companies should establish rigorous audit processes to identify and mitigate biases throughout the algorithm’s lifecycle.
Google’s Model Cards project demonstrates this approach well. It provides detailed information about a model’s performance across different demographics, helping developers and users understand potential biases and limitations.
Prioritize Transparency and Accountability
Transparency in algorithm development and deployment builds trust and enables external scrutiny. Companies should:
- Publish clear documentation on how their algorithms work
- Provide explanations for algorithmic decisions when possible
- Establish channels for user feedback and concerns
The AI Ethics Board at Axon (formerly Taser) exemplifies accountability in action. This independent board provides guidance on the ethical development and use of AI in Axon’s products, ensuring that the company addresses potential biases and negative impacts.
Embrace Ongoing Learning and Adaptation
The creation of inclusive algorithms requires continuous effort and adaptation as technology and society evolve. Companies must commit to ongoing learning, staying informed about new research and best practices in algorithmic fairness.
Regular training sessions for development teams on bias recognition and mitigation techniques can help maintain a focus on inclusivity throughout the development process.
Final Thoughts
Inclusive algorithms shape our digital experiences and impact real-world outcomes. These algorithms influence everything from hiring practices to criminal justice systems, underscoring their importance in creating fair digital spaces. Tech companies and developers must prioritize diverse data collection, interdisciplinary collaboration, and rigorous auditing processes to create algorithms that reflect our global community’s diversity.
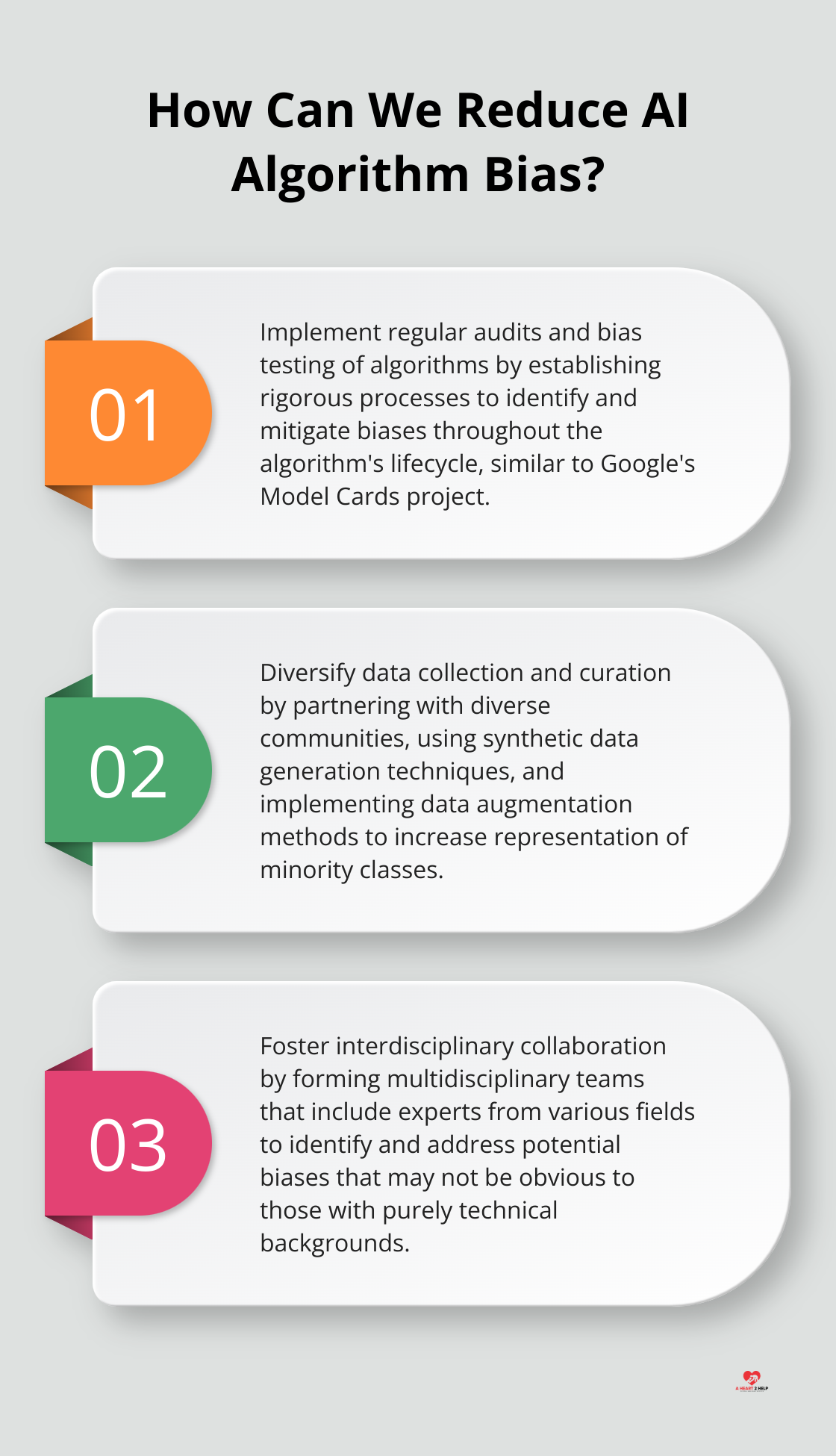
Transparency and accountability stand at the forefront of efforts to build trust with users and enable continuous improvement. A Heart 2 Help commits to leveraging technology for positive change in communities worldwide. Our care-app connects those in need with compassionate volunteers, using advanced matching algorithms to facilitate real-time support.
The path to truly inclusive algorithms presents challenges, but we must pursue it. We embrace this opportunity to create digital spaces that are fair, equitable, and reflective of our diverse world. Together, we can harness technology’s power to build a more inclusive digital future for all.